VIFNet: Visible-Infrared Fusion for Image Dehazing
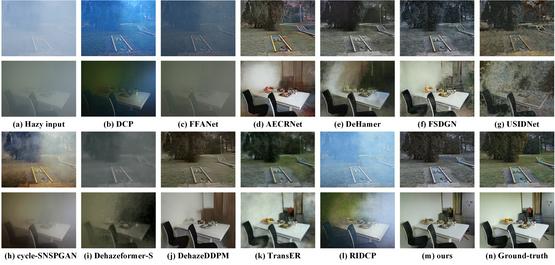
Key Takeaways from VIFNet
- VIFNet addresses the issue of information loss in dense haze scenarios that affect deep learning-based dehazing methods.
- The network uses a multi-scale Deep Structure Feature Extraction module with a Channel-Pixel Attention Block to improve information retention.
- VIFNet introduces an inconsistency weighted fusion strategy that leverages reliable information from both visual and infrared modalities.
- Evaluated on AirSim-VID dataset, VIFNet shows superior performance over other competing methods in realistic and simulated datasets.
Why is VIFNet Important?
VIFNet demonstrates how multimodal data fusion can significantly improve the performance of image processing tasks such as dehazing. By considering both visible and infrared information, the system ensures more robust performance in challenging environmental conditions. This research opens doors for further exploration of multimodal fusion techniques in various AI applications, such as autonomous driving and environmental monitoring. Read more.
Personalized AI news from scientific papers.