The Codecfake Dataset and Countermeasures for Universally Detecting Deepfake Audio
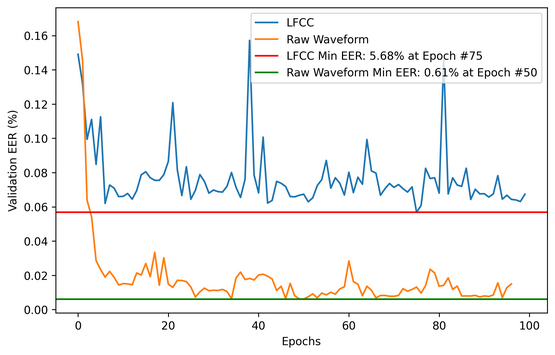
Abstract
Audio Language Models (ALMs) are evolving, and with their advancements, new challenges in detecting deepfake audio arise. This paper introduces Codecfake, a dataset designed specifically for training and evaluating deepfake audio detection models.
Key Findings:
- The dataset includes diverse examples across two languages to train robust detection models.
- The introduction of domain adaptive techniques to manage the discrepancy in training data for better generality across unseen data.
Implications:
- The development of more robust detection systems could help in early detection and prevention of audio-based misinformation.
- Training on such specifically crafted datasets could set new standards for benchmarking deepfake detection models.
Opinion:
This study underscores the critical need for focused efforts on developing tailored datasets for emerging threats like deepfake audio. The methods and dataset presented could serve as a blueprint for future research in multimedia security.
Personalized AI news from scientific papers.