Skeletal Action Recognition
Simba: Mamba augmented U-ShiftGCN for Skeletal Action Recognition in Videos
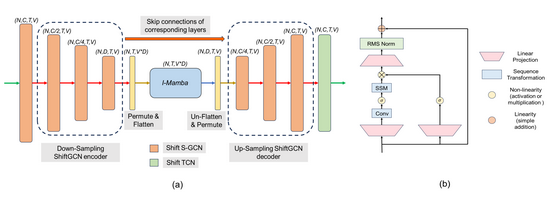
Summary & Takeaways
- Simba introduces a fresh take on Skeletal Action Recognition (SAR) by incorporating the Mamba component, an emerging alternative to attention mechanisms in Transformers.
- Constructs a U-ShiftGCN model with an encoder-decoder structure enhanced by Mamba for spatial and temporal feature extraction, offering great performance gains.
- Outstrips existing GCN-based and Transformer approaches on SAR tasks across multiple datasets.
Key Features:
- Enhanced Spatial Features: Extracts and refines spatial information for accurate action representation.
- Temporal Dynamics: Mamba block integrated to better capture action progression over time.
- Model Efficiency: Advanced architecture yielding superior performance with managed computational overhead.
Opinion
Combining Mamba and U-ShiftGCN is a promising direction for SAR and other time-series analyses, proving that careful architectural choices can lead to significant benefits. Simba’s results could inspire more effective human-robot interaction models.
Discover full details: Simba on arXiv
Personalized AI news from scientific papers.