Personalized LLM Fine-tuning
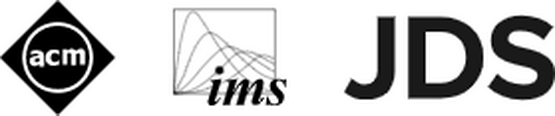
The study Democratizing Large Language Models via Personalized Parameter-Efficient Fine-tuning introduces a novel concept in LLM customization for enhanced user alignment.
- Personalization is achieved through tailored prompt design, profile-based retrieval, and behavioral history analysis.
- The method overcomes constraints posed by the lack of model ownership and privacy concerns in prior approaches.
- It integrates parametric and non-parametric knowledge to capture dynamic user behavior efficiently.
- OPPU (One PEFT Per User) utilizes personalized fine-tuning modules, offering users model ownership and a high degree of customization.
- The approach outperforms existing methods in handling behavior patterns, robustness, and versatility across tasks.
This research heralds a significant step in LLM personalization, emphasizing the model’s adaptability to individual preferences, behavior changes, and maintaining robustness. It emphasizes the need for personalized AI experiences and opens doors for further innovation in user-centric computing.
Personalized AI news from scientific papers.