Medical Image Segmentation
Mastering Medical Image Segmentation with Swin-UMamba
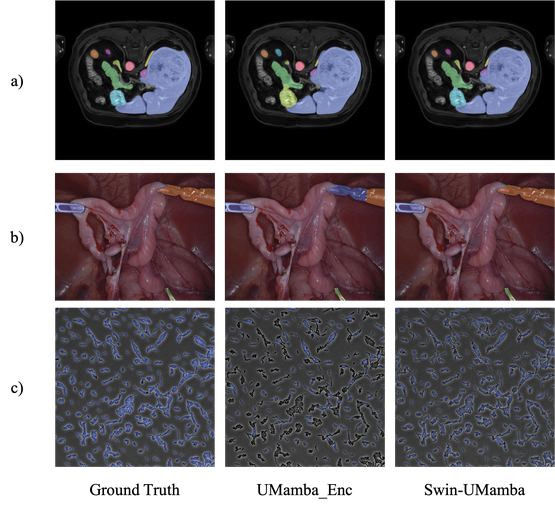
Summarizing ‘Swin-UMamba: Mamba-based UNet with ImageNet-based pretraining’
This study presents Swin-UMamba, blending the power of ImageNet pretraining with Mamba-based models to handle medical image segmentation. Profound takeaways:
- Multi-scale information integration is paramount for precise segmentation, and Swin-UMamba addresses this by leveraging long-range global info more efficiently.
- Pretraining on ImageNet significantly boosts performance, evidencing its importance in medical image analysis.
- Swin-UMamba shows an average improvement of 3.58% over traditional models across various datasets, illustrating its prowess in medical imaging.
With open-source availability, Swin-UMamba sets a new benchmark in the field and represents a titan leap forward in medical image processing and analysis.
Authors: Jiarun Liu, Hao Yang, et al.
Read the full paper on arXiv
Personalized AI news from scientific papers.