LLM-Driven Improvement in Negotiation Skills
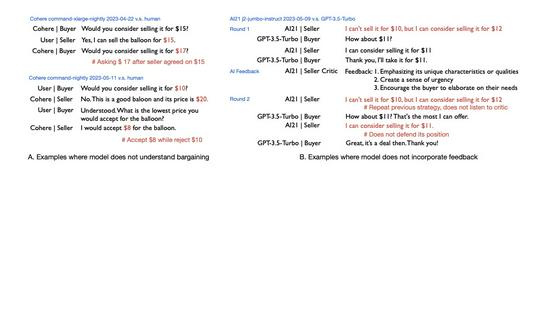
Summary
Innovative research explores the potential of Large Language Models (LLMs) to autonomously improve each other through a negotiation game involving roles as a buyer and a seller, augmented by a third model acting as a critic. The feedback from the critic model is utilized to enhance the negotiation strategies of the participating LLMs.
Key Findings
- Demonstration of negotiation improvements in LLMs through self-play, reflection, and the inclusion of AI feedback.
- Varying degrees of model competency based on role-play, revealing the challenges LLMs face when positioned differently within the negotiation scenario.
- Enhanced negotiation outcomes, with stronger agents showing significant improvements when iterative feedback is integrated into their strategy.
Further Insights
- The insights gained could lead to the development of robust AI-powered agents capable of complex interpersonal scenarios without human oversight. This could revolutionize fields like customer support, diplomacy, and business negotiations.
Opinion
This research is significant as it opens up possibilities for AI systems to train and improve autonomously, minimizing human inputs and showcasing potential practical applications in various industries. The findings could serve as a foundation for creating more sophisticated and capable AI negotiators.
Personalized AI news from scientific papers.