Graph Chain-of-Thought: Augmenting Large Language Models by Reasoning on Graphs
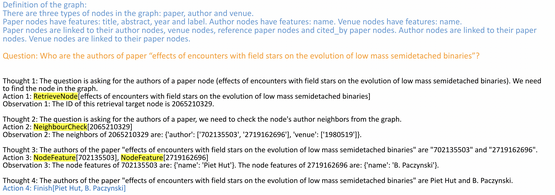
Explore the research
The ‘Graph Chain-of-Thought’ (Graph-CoT) framework aims to enhance the performance of large language models (LLMs) by incorporating reasoning over graphs depicting various domains. The framework systematically facilitates higher reasoning capabilities in LLMs through iterative graph interactions, leading to improved knowledge integration and processing. Highlights include:
- Integration of Graphical Data: Graph-CoT harnesses the power of interconnected texts and their relationships, forming knowledge graphs for better comprehension.
- Iterative Reasoning Enhancements: Each cycle of reasoning involves LLM interaction with the graph, enriching the model’s understanding and decision-making processes.
- Benchmarking Successes: The systematic experiments conducted with this framework show consistent outperformance over traditional methods, validating the effectiveness of graph-augmented reasoning strategies.
Personalized AI news from scientific papers.