GenEval: A Novel Framework for Evaluating Text-to-Image Models
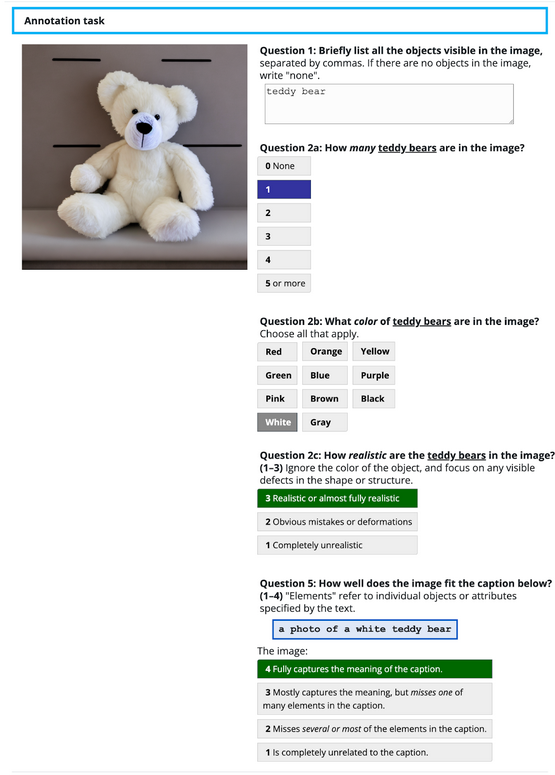
Evaluating Generative Models with GenEval
With the surge of text-to-image generative models, evaluating their performance has become a complex task. GenEval addresses this issue by focusing on object-related properties like co-occurrence, position, count, and color in images.
Main Insights:
- GenEval serves as an automated evaluation framework that allows for instance-level analysis, overcoming the limitations of holistic metrics such as FID or CLIPScore.
- By leveraging current object detection models, GenEval can assess text-to-image models with high human agreement.
- Analysis of open-source text-to-image models using GenEval has highlighted significant improvements in generative capabilities.
- However, these models struggle with complex tasks such as spatial relations and attribute binding.
Further Research Opportunities:
- Utilizing GenEval can reveal failure modes, guiding the development of the next generation of text-to-image models.
- Multimodal pretraining and efficient finetuning can benefit from GenEval to fine-tune generative performances.
In my opinion, GenEval is a crucial framework for the progression of generative AI, as it allows developers to pinpoint and rectify specific weaknesses in current models. The potential applications in various fields, ranging from design to automated content generation, underscore its importance.
Personalized AI news from scientific papers.