Retrieval-Augmented Generation
From Local to Global: A Graph RAG Approach to Query-Focused Summarization
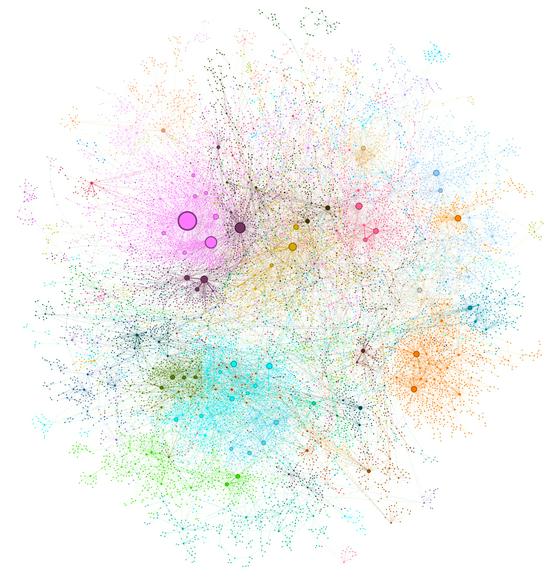
The new Graph RAG approach addresses the challenge of summarizing complex queries by intertwining RAG with structured graph models. This enhances the summarization capabilities of LLMs by allowing them to handle more general and voluminous data effectively.
- Technology Fusion: Merging RAG with graph theory to manage complex queries.
- Efficiency: Improved summarization for large, diverse text corpora.
- Methodology: Dual stage graph-based index creation leading to comprehensive summaries.
- Practical Applications: Potential use in real-world information retrieval and decision-making scenarios.
- Future Directions: Shows promise for further refining interactions between retrieval systems and generative models.
Graph RAG demonstrates a novel pathway for combining established AI methodologies to significantly enhance the flexibility and utility of language models for complex summarization tasks.
Personalized AI news from scientific papers.