Explainable Concept Drift for Cybersecurity
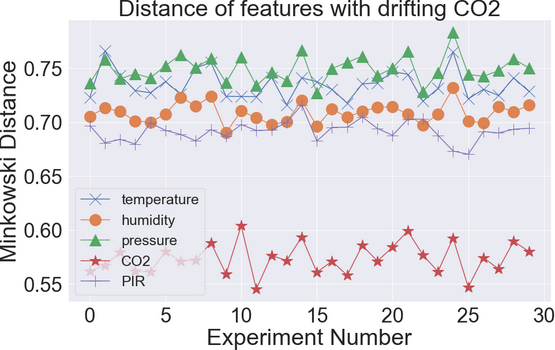
Autonomous systems, such as HVAC systems in smart buildings, are vulnerable to cybersecurity threats, which is the focus of the paper titled ‘Thwarting Cybersecurity Attacks with Explainable Concept Drift.’
- The study introduces a Feature Drift Explanation (FDE) module aimed at identifying features that cause drift in machine learning models due to cyber attacks.
- The method utilizes Auto-encoders to find latent representations of data and measures divergence using Minkowski distance.
- Experimentation shows that FDE can successfully pinpoint up to 85.77% of the drifting features, aiding in the adaptation of Deep Learning models under drift conditions.
- The proposed technique offers a promising direction for enhancing security measures against attacks that manipulate sensor data.
This research contributes significantly to the arsenal of tools available for protecting autonomous systems from cyber threats, offering a path towards greater systemic resilience.
By enabling a better understanding of drifting features, this method opens up new possibilities for real-time threat detection and response within smart environments.
Personalized AI news from scientific papers.