Deep Learning and LLM-based Methods Applied to Stellar Light Curve Classification
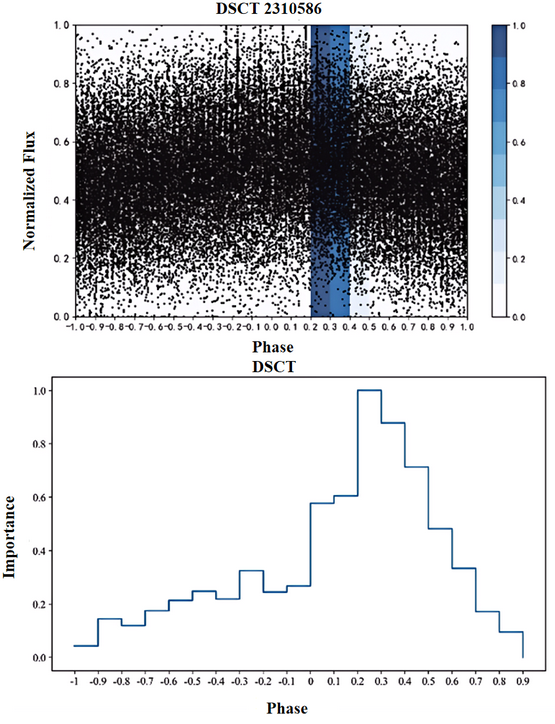
Explore the seamless integration of deep learning and LLM techniques to classify variable star light curves from the Kepler and K2 missions with commendable precision. This study highlights:
- The use of 1D-Convolution+BiLSTM architectures
- Swin Transformer applications
- Novel LLMs tailored for astronomical data
Accomplishments:
- Achieved accuracies up to 99% on selective models
- Recognized elusive Type II Cepheids with 83% accuracy
- Developed StarWhisper LightCurve, an LLM-based system for enhanced astronomy analysis
Further Details:
- The session revolves around the innovative application of LLMs ensuring minimal need for manual feature engineering thus enhancing efficiency.
- This system potentially opens new avenues for autonomous astronomical research, reducing human oversight and enabling faster, more accurate observations.
For more insightful analysis, view th… Challenges in multimodal model editing highlighted.
- MMEdit benchmark as a tool for pursuing further research in this domain.
Find out more at the complete paper.
This research not only provides a groundbreaking method for astronomic classifications but also underscores the growing relevance of LLMs in scientific domains beyond just language processing, paving the way for broader applications in complex, data-intensive fields.
Personalized AI news from scientific papers.