Aligning LLMs with Human Preferences via RLHF
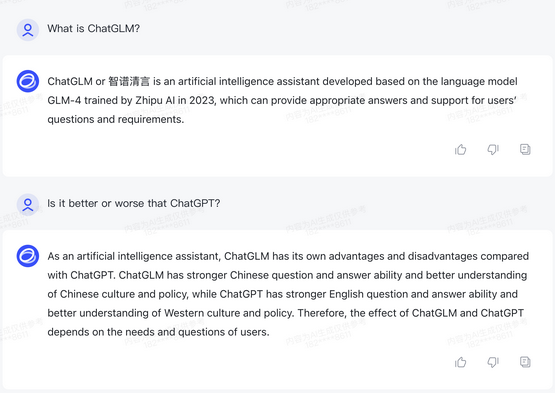
The paper ChatGLM-RLHF: Practices of Aligning Large Language Models with Human Feedback elucidates how the ChatGLM-RLHF pipeline significantly improves the alignment of LLMs with human preferences.
- Discusses the collection of human preference data and training of the reward model.
- Addresses challenges in implementing RLHF, such as reward variance and catastrophic forgetting.
- Demonstrates improvements in alignment tasks, with up to 15% more wins than previous models.
- Provides valuable insights into RLHF implementations in real-world LLM applications.
The success of ChatGLM-RLHF highlights the importance of human-centric AI development and offers robust strategies crucial for creating LLMs that are more attuned to human values and preferences.
Personalized AI news from scientific papers.